Launch this notebook on on mybinder.org:
Transport Map from density#
The objective of this example is to show how a transport map can be build in MParT when the the unnormalized probability density function of the target density is known.
Problem description#
We consider \(T(\mathbf{z};\mathbf{w})\) a monotone triangular transport map parameterized by \(\mathbf{w}\) (e.g., polynomial coefficients). This map which is invertible and has an invertible Jacobian for any parameter \(\mathbf{w}\), transports samples \(\mathbf{z}^i\) from the reference density \(\eta\) to samples \(T(\mathbf{z}^i;\mathbf{w})\) from the map induced density \(\tilde{\pi}_\mathbf{w}(\mathbf{z})\) defined as:
where \(\text{det } T^{-1}\) is the determinant of the inverse map Jacobian at the point \(\mathbf{z}\). We refer to \(\tilde{\pi}_{\mathbf{w}}(\mathbf{x})\) as the map-induced density or pushforward distribution and will commonly interchange notation for densities and measures to use the notation \(\tilde{\pi} = T_{\sharp} \eta\).
The objective of this example is, knowing some unnormalized target density \(\bar{\pi}\), find the map \(T\) that transport samples drawn from \(\eta\) to samples drawn from the target \(\pi\).
Imports#
First, import MParT and other packages used in this notebook. Note that it is possible to specify the number of threads used by MParT by setting the KOKKOS_NUM_THREADS
environment variable before importing MParT.
[1]:
import numpy as np
from scipy.optimize import minimize
import matplotlib.pyplot as plt
from scipy.stats import multivariate_normal
import os
os.environ['KOKKOS_NUM_THREADS'] = '8'
import mpart as mt
print('Kokkos is using', mt.Concurrency(), 'threads')
plt.rcParams['figure.dpi'] = 110
Kokkos::OpenMP::initialize WARNING: You are likely oversubscribing your CPU cores.
process threads available : 4, requested thread : 8
Kokkos::OpenMP::initialize WARNING: You are likely oversubscribing your CPU cores.
Detected: 4 cores per node.
Detected: 1 MPI_ranks per node.
Requested: 8 threads per process.
Kokkos is using 8 threads
Target density and exact map#
In this example we use a 2D target density known as the banana density where the unnormalized probability density, samples and the exact transport map are known.
The banana density is defined as:
where \(N_1\) is the 1D standard normal density.
The exact transport map that transport the 2D standard normal density to \(\pi\) is known as:
Contours of the target density can be visualized as:
[2]:
# Unnomalized target density required for objective
def target_logpdf(x):
rv1 = multivariate_normal(np.zeros(1),np.eye(1))
rv2 = multivariate_normal(np.zeros(1),np.eye(1))
logpdf1 = rv1.logpdf(x[0])
logpdf2 = rv2.logpdf(x[1]-x[0]**2)
logpdf = logpdf1 + logpdf2
return logpdf
# Grid for plotting
ngrid=100
x1_t = np.linspace(-3,3,ngrid)
x2_t = np.linspace(-3,7.5,ngrid)
xx1,xx2 = np.meshgrid(x1_t,x2_t)
xx = np.vstack((xx1.reshape(1,-1),xx2.reshape(1,-1)))
# Target contours
target_pdf_at_grid = np.exp(target_logpdf(xx))
fig, ax = plt.subplots()
CS1 = ax.contour(xx1, xx2, target_pdf_at_grid.reshape(ngrid,ngrid))
ax.set_xlabel(r'$x_1$')
ax.set_ylabel(r'$x_2$')
h1,_ = CS1.legend_elements()
legend1 = ax.legend([h1[0]], ['target density'])
plt.show()
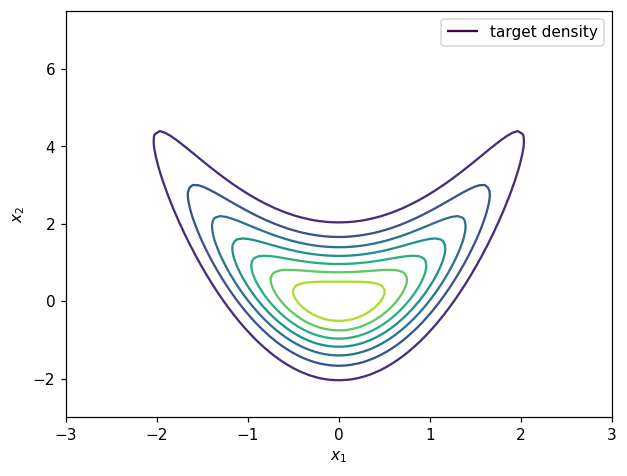
Map training#
Defining objective function and its gradient#
Knowing the closed form of the unnormalized target density \(\bar{\pi}\), the objective is to find a map-induced density \(\tilde{\pi}_{\mathbf{w}}(\mathbf{z})\) that is a good approximation of the target \(\pi\).
In order to characterize this posterior density, one method is to build a monotone triangular transport map \(T\) such that the KL divergence \(D_{KL}(\eta || T^\sharp \pi)\) is minmized. If \(T\) is map parameterized by \(\mathbf{w}\), the objective function derived from the discrete KL divergence reads:
where \(T\) is the transport map pushing forward the standard normal \(\mathcal{N}(\mathbf{0},\mathbf{I}_d)\) to the target density \(\pi(\mathbf{z})\). The gradient of this objective function reads
The objective function and gradient can be defined using MParT as:
[3]:
# KL divergence objective
def obj(coeffs, transport_map, x):
num_points = x.shape[1]
transport_map.SetCoeffs(coeffs)
map_of_x = transport_map.Evaluate(x)
logpdf= target_logpdf(map_of_x)
log_det = transport_map.LogDeterminant(x)
return -np.sum(logpdf + log_det)/num_points
# Gradient of unnomalized target density required for gradient objective
def target_grad_logpdf(x):
grad1 = -x[0,:] + (2*x[0,:]*(x[1,:]-x[0,:]**2))
grad2 = (x[0,:]**2-x[1,:])
return np.vstack((grad1,grad2))
# Gradient of KL divergence objective
def grad_obj(coeffs, transport_map, x):
num_points = x.shape[1]
transport_map.SetCoeffs(coeffs)
map_of_x = transport_map.Evaluate(x)
sens_vecs = target_grad_logpdf(map_of_x)
grad_logpdf = transport_map.CoeffGrad(x, sens_vecs)
grad_log_det = transport_map.LogDeterminantCoeffGrad(x)
return -np.sum(grad_logpdf + grad_log_det, 1)/num_points
Map parameterization#
For the parameterization of \(T\) we use a total order multivariate expansion of hermite functions. Knowing \(T^\text{true}\), any parameterization with total order greater than one will include the true solution of the map finding problem.
[4]:
# Set-up first component and initialize map coefficients
map_options = mt.MapOptions()
total_order = 2
# Create dimension 2 triangular map
transport_map = mt.CreateTriangular(2,2,total_order,map_options)
Approximation before optimization#
Coefficients of triangular map are set to 0 upon creation.
[5]:
# Make reference samples for training
num_points = 10000
z = np.random.randn(2,num_points)
# Make reference samples for testing
test_z = np.random.randn(2,5000)
# Pushed samples
x = transport_map.Evaluate(test_z)
# Before optimization plot
plt.figure()
plt.contour(xx1, xx2, target_pdf_at_grid.reshape(ngrid,ngrid))
plt.scatter(x[0],x[1], facecolor='blue', alpha=0.1, label='Pushed samples')
plt.legend()
plt.show()
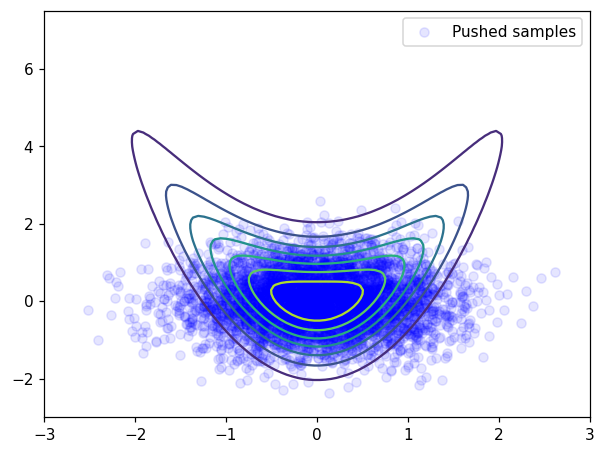
At initialization, samples are “far” from being distributed according to the banana distribution.
Initial objective and coefficients:
[6]:
# Print initial coeffs and objective
print('==================')
print('Starting coeffs')
print(transport_map.CoeffMap())
print('Initial objective value: {:.2E}'.format(obj(transport_map.CoeffMap(), transport_map, test_z)))
print('==================')
==================
Starting coeffs
[0. 0. 0. 0. 0. 0. 0. 0. 0.]
Initial objective value: 3.40E+00
==================
Minimization#
[7]:
print('==================')
options={'gtol': 1e-4, 'disp': True}
res = minimize(obj, transport_map.CoeffMap(), args=(transport_map, z), jac=grad_obj, method='BFGS', options=options)
# Print final coeffs and objective
print('Final coeffs:')
print(transport_map.CoeffMap())
print('Final objective value: {:.2E}'.format(obj(transport_map.CoeffMap(), transport_map, test_z)))
print('==================')
==================
Optimization terminated successfully.
Current function value: 2.848868
Iterations: 17
Function evaluations: 19
Gradient evaluations: 19
Final coeffs:
[ 1.64386417e-02 8.40185180e-01 1.11002314e-02 9.88287812e-01
8.46815229e-01 -7.30824747e-03 7.15717444e-02 -1.61399131e-03
2.21101864e+00]
Final objective value: 2.83E+00
==================
Approximation after optimization#
Pushed samples#
[8]:
# Pushed samples
x = transport_map.Evaluate(test_z)
# After optimization plot
plt.figure()
plt.contour(xx1, xx2, target_pdf_at_grid.reshape(ngrid,ngrid))
plt.scatter(x[0],x[1], facecolor='blue', alpha=0.1, label='Pushed samples')
plt.legend()
plt.show()
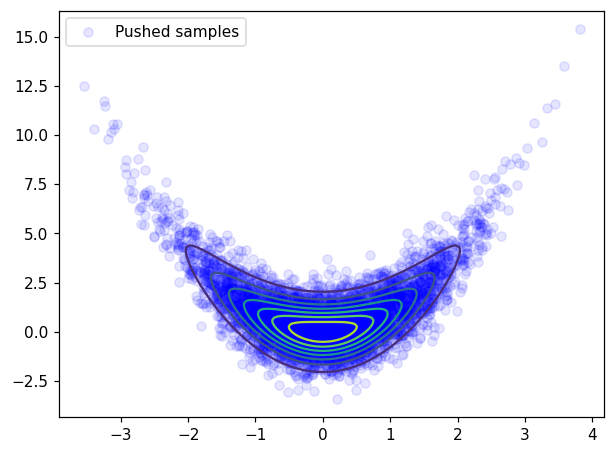
After optimization, pushed samples \(T(z^i)\), \(z^i \sim \mathcal{N}(0,I)\) are approximately distributed according to the target \(\pi\)
Variance diagnostic#
A commonly used accuracy check when facing computation maps from density is the so-called variance diagnostic defined as:
This diagnostic is asymptotically equivalent to the minimized KL divergence \(D_{KL}(\eta || T^\sharp \pi)\) and should converge to zero when the computed map converge to the true map.
The variance diagnostic can be computed as follow:
[9]:
def variance_diagnostic(tri_map,ref,target_logpdf,x):
ref_logpdf = ref.logpdf(x.T)
y = tri_map.Evaluate(x)
pullback_logpdf = target_logpdf(y) + tri_map.LogDeterminant(x)
diff = ref_logpdf - pullback_logpdf
expect = np.mean(diff)
var = 0.5*np.mean((diff-expect)**2)
return var
[10]:
# Reference distribution
ref_distribution = multivariate_normal(np.zeros(2),np.eye(2));
# Compute variance diagnostic
var_diag = variance_diagnostic(transport_map,ref_distribution,target_logpdf,test_z)
# Print variance diagnostic
print('==================')
print('Variance diagnostic: {:.2E}'.format(var_diag))
print('==================')
==================
Variance diagnostic: 3.34E-04
==================
Pushforward density#
We can also plot the contour of the unnormalized density \(\bar{\pi}\) and the pushforward approximation \(T_\sharp \eta\):
[11]:
# Pushforward definition
def push_forward_pdf(tri_map,ref,x):
xinv = tri_map.Inverse(x,x)
log_det_grad_x_inverse = - tri_map.LogDeterminant(xinv)
log_pdf = ref.logpdf(xinv.T)+log_det_grad_x_inverse
return np.exp(log_pdf)
map_approx_grid = push_forward_pdf(transport_map,ref_distribution,xx)
fig, ax = plt.subplots()
CS1 = ax.contour(xx1, xx2, target_pdf_at_grid.reshape(ngrid,ngrid))
CS2 = ax.contour(xx1, xx2, map_approx_grid.reshape(ngrid,ngrid),linestyles='--')
ax.set_xlabel(r'$x_1$')
ax.set_ylabel(r'$x_2$')
h1,_ = CS1.legend_elements()
h2,_ = CS2.legend_elements()
legend1 = ax.legend([h1[0], h2[0]], ['Unnormalized target', 'TM approximation'])
plt.show()
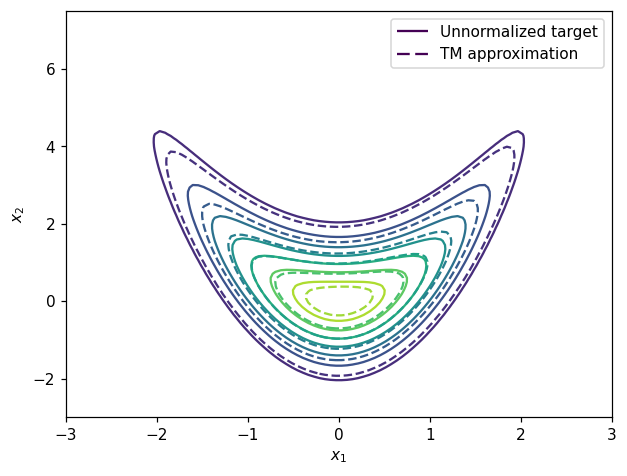
[ ]: